Building on the disruption unleashed by Gen-AI in the past year, the rapid rate of innovation in AI and cloud-native tech solutions, will see an explosion in data in the near future. By 2025, global data volume is expected to reach 175 zetabytes and experts predict that data will be embedded in every decision, interaction and process.
Challenges of the data overload : indecision and inaction
With companies operating in complex data environments with various technologies working independently, it can be challenging to achieve scalable digital transformation due to legacy architecture and talent skill gaps. As a result, businesses may use technology solutions without fully understanding their business context or relying on incorrect metrics, eventually leading to information overload. This, in turn, can cause indecisiveness and inaction, exposing companies to potential threats and making it difficult to identify and act on scalable opportunities. Additionally, it can also lead to difficulties in managing risk.
Unlocking value through distributed and scalable data operations
Top of mind for business leaders is finding ways to maximise value from enterprise data, empowering their team’s decision-making and improving business resiliency. This is why the Human Managed I.DE.A modular, cloud-native platform was developed – enabling businesses to operationalise data from any source and make smarter decisions and faster actions for better cyber, digital and risk outcomes. The platform adapts to every business context through 3 offerings –
- Data Ops – Distributed data engineering as input for AI models – This ensures that whatever data a business needs to analyse, is continuously collected, processed and stored to be AI-ready.
- ML Ops – Tuning AI models personalised for each business – This ensures that AI models are trained, tuned and improved with data, logic, and patterns unique to each company.
- Intel Ops – Getting the right intel to inform the right decision and action at the right time – this involves ensuring that DataOps and MLOps within a business are working in tandem in a consistent and scalable operational cycle across the enterprise.
Data Ops : Collect, receive and prepare any data from any source
The Human Managed DataOps platform allows any data to be connected on demand. The associated microservices make the platform data-agnostic and tool-agnostic. We can collect and receive enterprise data and prepare it for analysis, no matter the size, form or location.
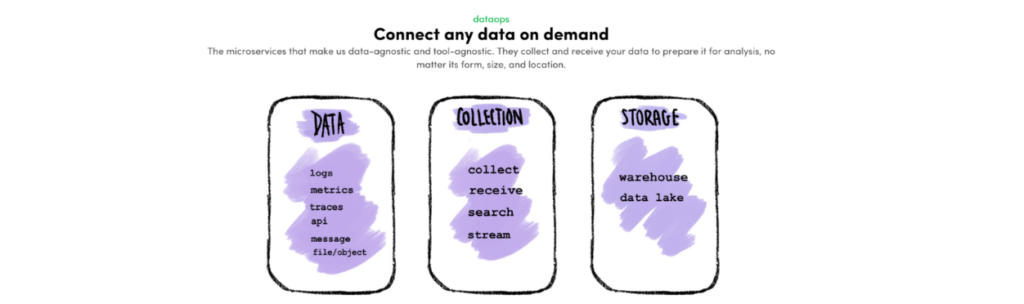
Case study for Human Managed Data Ops
We were approached by a leading ASEAN conglomerate, to help them with a
a widely shared problem in cyber operations: effective prioritisation. Our client had grappled with siloed asset databases for over two decades. Managing disparate cybersecurity tools across public and vendor clouds, along with on-premise systems, led to manual and sluggish operations, resulting in overlooked issues.
The goal was to automatically contextualise and prioritise cybersecurity concerns in real-time. The client chose ten key data sources that provided us with required inputs [alerts, logs, metrics from SaaS and on-prem systems] and context (asset databases, strategies, and business logic).
The customer’s data was onboarded onto the HM platform for continuous cyber operations in less than a month. We catalogued their assets, controls and attributes and structured their cybersecurity alerts, logs and metrics under one data schema and model. Our solution meant that the variable components for any analysis by any kind of computing, whether rule-based programming or AI/ML models, were ready.
ML Ops : Tuning foundation AI models unique to business context
AI models, whether open or commercial, undergo training on generalised and unlabelled data, often sourced from the Internet or untraceable databases. Despite their sophistication, generic AI models do not inherently generate accurate and precise outputs tailored to a specific business context. Achieving operational effectiveness in machine learning requires the customization of AI models through training, tuning, and improvement using data, logic, and patterns specific to each business. This personalised approach is essential for creating foundational AI models that align with the unique requirements and nuances of a specific business environment.
The Human Managed MLOps platform tunes foundation AI models with a knowledge base that is unique to each customer’s business context and incorporates a human-in-the-loop feedback cycle to continuously improve and measure the performance of the customer’s personalised AI model. This way, our customers get the best of both worlds: trending knowledge that builds foundation models and tribal knowledge that builds their own context models.
Case study for Human Managed ML Ops –
In the case of a global banking customer, we apply MLOps for business-critical use case – fraud detection and management. Consistently tuning AI models using the bank’s personalised data allowed the creation of a contextualised fraud model. This model is adept at classifying, predicting, or indicating suspicious and potentially fraudulent activities.
Over years of operations, our client had accumulated valuable tribal knowledge and experiences related to fraud scenarios. They sought an automated and scalable solution to enhance detection accuracy and reduce response time in addressing fraudulent activities.
Examples of their tribal knowledge include indicators of different types of fraud (e.g. repeated withdrawals from the same account on the same day), when a detection alert should be triggered (e.g. withdrawal amount is higher than US$50,000), and what needs to be done when there is a detection (e.g. send a critical alert to fraud investigation unit to investigate suspicious transaction).
The repository of these data points constitutes our customer’s distinctive context, encompassing aspects like business logics, prioritised assets, and historical patterns. Through the Human Managed MLOps platform, these data points undergo transformation into structured data and code. This process results in the creation of features and labels that tune the customer’s personalised fraud model based on their unique context.
Upon aligning input data, AI/ML processes, and the desired output, a virtuous circle of human and machine collaboration ensues. This collaboration is continuous, as ML models enhance their capabilities with additional training and feedback. The customer consistently incorporates more datasets, rules, and conditions, while the platform continuously learns from this data to enhance the accuracy of their ransomware model.
Intel Ops: Serving intel to the right audience at the right time
Getting the proper intel consistently is a challenging feat. However, the benefits of the right intel are limited if you do not apply it to the right problem at the right time. Even with the best intel made available through DataOps and MLOps, if it is not served to the right audience at the right time, the value of that intel is not realised, and the window of opportunity closes. The challenge here is to bring DataOps and MLOps processes together in a consistent and scalable operational cycle across the business, which we call IntelOps. This is a collection of microservices for executing use cases to generate IDEAS. [Intelligence. Decision. Actions].
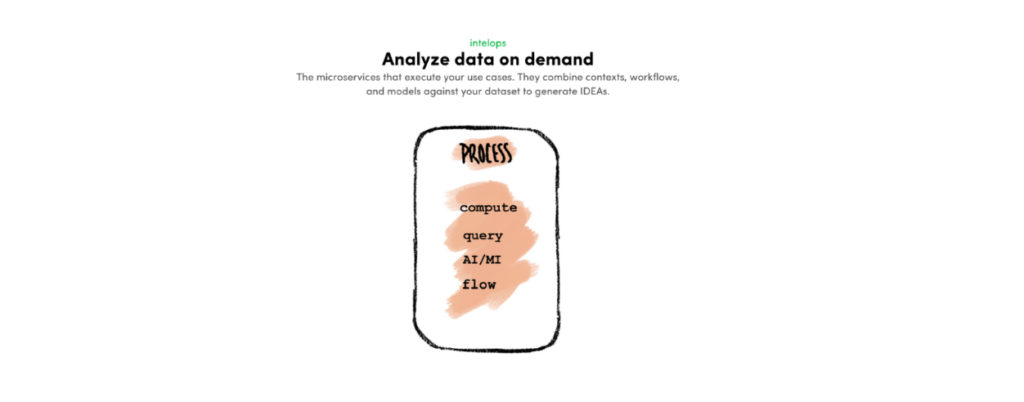
Case study for Human Managed Intel Ops –
One of our customers subscribed to our network security posture management service to move the needle on fixing 40,000 violations that have been open and unresolved for over two years. Contextualised intel on the violations — no matter how organised and easy to understand — did not get their operations team to decide on the next steps because the number of issues was so high.
To make progress on the cyber posture issue, the customer asked for decisions and actions that would have the “biggest bang for the buck”, which is precisely what we delivered. Our IntelOps platform ran computation on 40,000 reported violations on 1000 network segments, protecting around 50,000 assets accessed by 40,000 employees. As an output, we generated four ranked decisions and 16 prescriptive actions that would remove 40 percent of all violations and improve 100 per cent of customer’s critical assets. The prescriptive actions were delivered through a nudge-based dispatch system to the users who could affect the change.
In conclusion, operationalising enterprise data is all about being fast and agile to fix issues, leverage business opportunities and remain resilient and alert. By automating basic day-to-day operations, organisations can become better at decision making, while employees can focus on more strategic human domains such as collaboration and innovation. The road to operationalising data begins with fostering a data-driven culture across all functions and levels within a company.
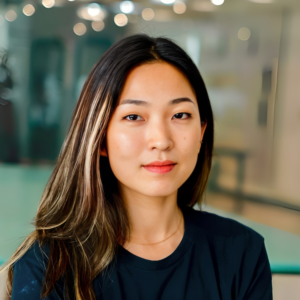
This article is written by Karen Kim, Chief Executive Officer, Human Managed
The insight is published as part of UPTECH MEDIA’s thought leadership piece, written within its repository of contributor articles.
UPTECH MEDIA welcomes partner article contributions about the latest technology trends in the Asia-Pacific region. For inquiries and submissions, please send them to [email protected].